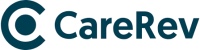
How CareRev Uses Comet MPM and Experiment Management to Accelerate Innovation
California-based healthcare startup CareRev knows machine learning is critical in improving customer experience and driving company growth. They function as a marketplace by connecting healthcare professionals with facilities and patients. The CareRev app allows users to select their preferred shifts and facilities, while healthcare facilities use CareRev as a SaaS to meet staffing demands.
The team at CareRev has been in a hyper-growth mode over the past few months. Even as a new machine learning team, their Machine Learning Engineers knew they needed an ML platform with a powerful UI to track and compare their experiments. They were looking for a tool to help their ML team scale, reduce costs, and provide data scientists with better insight into the model training process, saving them time. CareRev loves Comet’s efficiency and easy integration with other tools.
“The real selling point of Comet was how it could easily integrate with our training code, easily track everything that we needed, and have a good UI to show it when comparing experiments,” says Kes Crandall, ML Engineer at CareRev. After reviewing multiple vendors, the company ultimately decided to purchase Comet instead of another company or hire programmers to build something similar from scratch. “The cost of building something like Comet would have been incredibly high because it has an easy-to-use UI component and custom visualization features. And also, from a buying standpoint, it was pretty cheap for what the registry and experiment tracker offers. It was a no-brainer,” added Crandall.
A Central Repository for Faster Iteration
The CareRev team initially started with MLflow, an open-source experiment management platform that didn’t meet their needs. “It was not well maintained, and compared to Comet, it didn’t have all the features needed to track experiments, especially as we started scaling,” said Crandall.
Doubling back on experiments to see which model performed best can be frustrating. With no versioning or tracking, teams risk expending unnecessary effort on training runs. The CareRev team was looking for a solution that offered MPM, experiment tracking, and a model registry all in one tool to store their experiment results and remove some friction. With a central repository, they could compare results, visualize the data, and reproduce models. Implementing Comet’s platform helped them achieve just that. For instance, Comet’s Artifacts feature helped the CareRev team manage their assets and artifacts for easier reproducibility. “The assets and artifact section of Comet saved me a lot of time. Previously, whenever we were testing models, we had to go and find all the inputs we added to the model after viewing the model’s performance. But with Comet, they have all that stored for you. You can go to that singular link and grab all the model information you may want rather than going through a bunch of different services elsewhere to find that,” said Michael Fanous, an ML Engineer intern at CareRev.
Comet’s Features and Benefits
Machine learning is a highly iterative process that requires the diligent tracking of multiple sources of variability. Tracking changing variables becomes extremely difficult when dealing with multiple experiments simultaneously. An experiment management tool like Comet makes model development more repeatable and transparent by organizing your entire workflow from start to finish. When asked about their favorite Comet feature, Alec Karfonta, a Senior ML Engineer of CareRev, said that “it’s the big table of all of the experiments where I can select which columns to show up so I can see the different hyperparameters for the model, the different metrics that we want to look at, and just having those all together in one place is a game changer.” Fanous also responded that his favorite Comet feature was “the tagging system where you can tag different experiments based on different things you’re trying to try out with the model. When you do that, it’s really easy to aggregate the data in a way in which analysis is much simpler versus going run for run and checking every single outcome.”
One of the most significant benefits of a central ML repository is that it helps your team collaborate more efficiently and consistently. “Comet helped us collaborate more easily with one another. And having that trusted source for our test data that we could always go to and make sure it was running the same way when we were reporting results against each other, it’s super useful,” said Karfonta.
Since using Comet, CareRev has significantly increased saved time and productivity. The first model they released before using Comet was performing differently than expected. And so, they trained a new model for comparison, calculated feature importance, and used other evaluation tools to select the best one. From there, they were able to push the best model quickly. “From training to pushing the new model out, it took us a couple of hours because of the CI/CD pipeline we integrated with the [model] registry. Everything was just super seamless after we integrated with Comet. So that could have been a one or day two thing if we didn’t have Comet,” says Crandall.
The Icing on the Cake: Comet’s Customer Support
Most companies rely on email when communicating with their customers for technical support. However, it has several drawbacks, including a lag in response times, lack of a personal touch, ignored messages, and potential misunderstandings. “Email is just kind of ugly. It’s hard to talk to someone. It’s hard to follow a conversation. Slack is just the obvious form, and it’s just how you communicate with people generally. It’s easier to communicate with support that way,” says Crandall.
Comet’s Slack Community was one of the things that made the onboarding and implementation process for CareRev friction-free. When CareRev’s ML team encounters roadblocks, they can easily message the Comet team through Slack. “They were pretty responsive on Slack during implementation,” says Crandall.
The Comet team’s responsiveness during implementation is just one of the many things that the CareRev team appreciates about Comet’s customer service. “I like how easy it is to request new features on Slack and how receptive people are to understanding them. They update you on the roadmap and what’s coming so you can plan for it,” Crandall says.
“They’ve even asked us for what we would think would be good new features, and they’ve put that in their schedule, so that’s really cool,” agrees Karfonta. When asked about advising ML teams considering Comet, Karfonta says, “Comet covers about 90% of our needs. The last 10% is really niche stuff that’s only for us, and we’ve had to build out custom, but it’s been pretty good so far.”
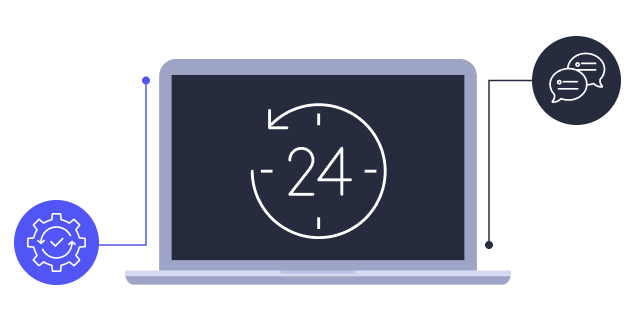