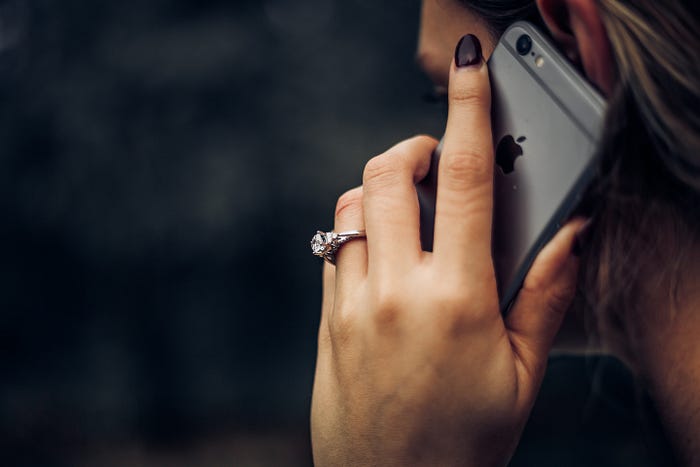
Introduction
Have you realized how rapidly artificial intelligence and machine learning have developed over the past few years? Machine learning algorithms can process and analyze enormous volumes of data, which enables them to grow and learn over time. Various sectors, including healthcare, banking, and manufacturing, stand to benefit from the integration of human and machine learning.
However, ensuring the algorithms are transparent and ethical is one of the most significant difficulties. The outcomes of machine learning algorithms may be biased or unexpected if they are not carefully developed and maintained. Machine learning includes speech recognition as a crucial element. This article will describe how RoBERTa can be used to recognize speech.
Speech Recognition
Speech recognition involves converting spoken language into text so that computers can hear and interpret it more easily. Numerous applications, such as automated customer service, virtual assistants, and speech-to-text transcription, use speech recognition extensively.
One of the most popular techniques for speech recognition is natural language processing (NLP), which entails training machine learning models on enormous amounts of text data to understand linguistic patterns and structures.
The RoBERTa model has recently emerged as a powerful tool for NLP tasks, including speech recognition.
RoBERTa
RoBERTa (Robustly Optimized BERT Approach) is a natural language processing (NLP) model based on the BERT (Bidirectional Encoder Representations from Transformers) architecture. It was developed by Facebook AI Research and released in 2019. It is a state-of-the-art model for a variety of NLP tasks.
Why Did RoBERTa Get Developed?
- One of the main reasons for developing RoBERTa was to address the issue of the “pre-training and fine-tuning discrepancy.” This refers to the fact that BERT was pre-trained on one set of tasks but fine-tuned on a different set of tasks for downstream NLP applications. This discrepancy could lead to suboptimal performance on the fine-tuning tasks. It was pre-trained on a more extensive and diverse data set to address this.
- Another primary reason for developing RoBERTa was to improve the training process itself. A larger batch size was used during training, allowing for more efficient hardware use and faster training times. The model also achieved superior performance on various NLP tasks through longer training and a more robust approach than BERT.
Architecture of RoBERTa
RoBERTa’s architecture is based on the BERT (Bidirectional Encoder Representations from Transformers) architecture, with some modifications and improvements. The main components of the RoBERTa architecture are explained below.
- Transformer Blocks: Like BERT, RoBERTa uses a series of transformer blocks to process the input sequence. Each transformer block consists of multi-head self-attention layers and feed-forward layers. The self-attention layers allow the model to focus on different parts of the input sequence. In contrast, the feed-forward layers will enable it to learn nonlinear relationships between the input tokens.
- Pre-Training Objectives: Using a masked language modeling approach, RoBERTa is pre-trained to anticipate the input tokens that have been randomly masked based on the context. RoBERTa trains the model to assess whether two input sequences in a specific text corpus are contiguous through the “next sentence prediction” objective.
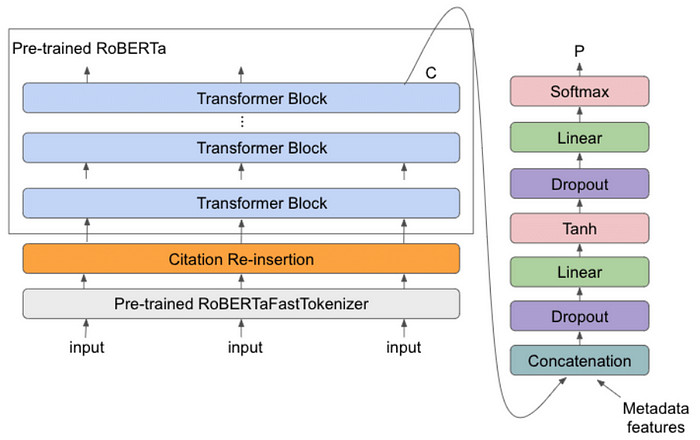
3. Pre-Processing: Before putting the text into the transformer blocks, pre-processing steps like byte pair encoding (BPE) and sentence piece tokenization segment the input text into smaller subwords, enabling the model to handle out-of-vocabulary (OOV) words.
4. Training Procedure: It is trained using a large corpus of text data, such as Wikipedia and Books Corpus. The training procedure involves training the model on multiple tasks and using a large batch size to improve efficiency. RoBERTa also uses a more robust training approach than BERT, including dynamic masking and no sentence-level segment embeddings.
Disadvantages using RoBERTa
RoBERTa differs from the original BERT model in several ways, including better training techniques, larger training datasets, and longer training timeframes. However, there are several drawbacks to employing RoBERTa that should be taken into account.
- Computational Resources: It is a very large model with over 320 million parameters. Training and using the model requires significant computational resources, including powerful GPUs and large amounts of memory. This makes it challenging for individuals and organizations without access to these resources to use the model effectively.
- Training Time: The RoBERTa model demands a significant amount of training time compared to less complex models. Training a RoBERTa model from scratch may take days to weeks on a single GPU and even longer on less powerful hardware. As a result, it is difficult for researchers and organizations to test and iterate on novel models quickly.
- Interpretability: Like many other deep learning models, RoBERTa is frequently referred to as a “black box.” This suggests that understanding how the model derives its predictions can be difficult and problematic in some applications.
- Overfitting: RoBERTa, like any deep learning model, is prone to overfitting. This can happen when the model becomes too complex or there is insufficient training data to generalize to new examples properly. While RoBERTa was designed to be more robust to overfitting than the original BERT model, it is still important to carefully tune the model’s hyperparameters and use appropriate regularization techniques to avoid overfitting.
- Pretrained-only: To train for a particular task, a lot of labeled data must be collected. Therefore, fine-tuning this model may not be particularly useful if the task doesn’t have enough labeled data or differs dramatically from the tasks it was pre-trained on.
Implementation
This section will discuss the implementation of speech recognition using RoBERTa. This code can perform speech recognition on an audio file.
Step 1: The most popular Python speech and audio analysis tool is SpeechRecognition, which can be installed using the command.
pip install SpeechRecognition
Step 2: It’s required to install the following libraries in your Python environment:
- PyTorch: A popular open-source machine learning framework for Python that can be used for building neural networks.
- Transformers: A Python library that provides pre-trained models for NLP tasks like text classification, question answering, and language generation.
- Sound device: A library for recording and playing sound with Python.
- Soundfile: A library for reading and writing sound files with Python.
import speech_recognition as sr
from transformers import RobertaTokenizer, RobertaForSequenceClassification
import torch
Step 3: Initialize the speech recognition recognizer.
recognizer = sr.Recognizer()
Step 4: Load the pre-trained RoBERTa model and tokenizer
model_name = "roberta-base"
tokenizer = RobertaTokenizer.from_pretrained(model_name)
model = RobertaForSequenceClassification.from_pretrained(model_name)
Step 5: Function to transcribe audio and perform RoBERTa processing
# Function to transcribe audio and perform RoBERTa processing
def transcribe_and_process_audio(audio_file_path):
with sr.AudioFile(audio_file_path) as source:
audio = recognizer.record(source) # Record the audio from the file
try:
# Perform speech recognition
transcription = recognizer.recognize_google(audio)
print("Transcription:", transcription)
# Process the transcription using RoBERTa
inputs = tokenizer(transcription, return_tensors="pt")
outputs = model(**inputs)
logits = outputs.logits
predicted_class = torch.argmax(logits, dim=1).item()
print("Predicted Class:", predicted_class)
# You can perform further processing on the transcribed text or the RoBERTa output as needed.
except sr.UnknownValueError:
print("Speech recognition could not understand audio")
except sr.RequestError as e:
print(f"Could not request results from Google Speech Recognition service; {e}")
Step 6: Provide the path to an audio file and start the process.
audio_file_path = "your_audio_file.wav"
transcribe_and_process_audio(audio_file_path)
Conclusion
Speech recognition has become an increasingly important technology in recent years, with applications in various fields, including medicine, education, and entertainment. In this article, we have explored how to transcribe audio using speech recognition and process with RoBERTa.
Due to its adaptability and scalability, the RoBERTa architecture is suitable for various voice recognition applications. Future research could focus on improving the accuracy and speed of voice recognition algorithms and looking into new uses for this technology.